7월 3주차 그래프 오마카세
Link prediction for ex ante influence maximization on temporal networks
[https://arxiv.org/pdf/2305.09965.pdf]

Introduction
SNS 다들 한번쯤은 사용해보셨을텐데요. 혹시 ‘언제 게시물을 포스팅해야 조회수와 같은 노출이 잘 될까?”라는 고민 해보시지 않으셨나요? 저는 어렸을 적 싸이월드에 다이어리 작성 , 일촌평 남김 과 같은 행위를 반복하며 자연스레 ‘학생들이 자주 보는 시간대’를 유추할 수 있게 되었고 그에 따라 많은 노출 , 관심을 받는데에 행복을 느꼈습니다. 지금 이 그래프 오마카세도 마찬가지로 ‘일요일 저녁 , 밤’이라는 시간대에 여러분들께서 관심을 가져주시기에 적절한 시간대라는 판단하에 매주 이맘때에 발송을 하는거구요.
서두에 작성했던 경험은 온전히 ‘감’에 의존하였는데요. 이 ‘감’을 계량화하여 언제 내 정보가 잘 전달될까 즉, 상대적으로 많이 증폭되어 다른 사람에게 전달될까 라는 것을 연구하는 분야가 있습니다. 바로 “influence maximization(IM)”입니다. 저희에게 가장 친근한 분야는 ‘소셜네트워크의 바이럴 마케팅’ ,’코로나 감염자 유추’ 이지 않을까 싶습니다.
본 논문은 앞서 언급한 IM technique 을 반영하여 link prediction 에 활용합니다. 어떤 노드를 seed node 로 설정하고 시간에 따라 seed node 의 영향력을 전달하고 , 전달받는 temporal network view 를 반영한다면 link prediction performance 가 향상될것이다 라는 가설을 검증하는게 본 논문의 골자라고 할 수 있습니다.
Preliminary
In the context of network science, the concept of "information maximization" can be related to the dissemination and utilization of information in a network. It pertains to maximizing the efficiency and effectiveness of information flow within a network. Here's an overview of information maximization in the context of network science:
- Diffusion and Spreading Models: Information maximization in network science involves studying how information, opinions, or behaviors spread through a network. Various diffusion and spreading models are used to understand and simulate this process, such as the epidemic models, influence maximization models, or rumor spreading models.
- Information Cascades: Information cascades refer to the propagation of information through a network where individuals adopt a particular behavior or belief based on the actions or decisions of their neighbors. Maximizing information in this context involves studying how to trigger large-scale cascades or to influence the behavior of individuals in a network to maximize the spread of information.
- Influence Maximization: Influence maximization is the task of identifying a small subset of influential nodes in a network that can maximize the spread of information or influence. The goal is to strategically select nodes to initiate information cascades and ensure the broadest dissemination of information within the network.
- Network Resilience: Information maximization also considers the resilience of a network to disruptions or attacks that can hinder the spread of information. Maximizing information flow involves studying strategies to enhance network robustness, identify critical nodes for information dissemination, or design efficient communication protocols.
- Optimal Routing: Information maximization can also be viewed in the context of optimizing the routing of information in a network. This involves finding the most efficient paths or routing strategies to maximize the throughput, minimize delays, or ensure reliable information transmission.
Overall, information maximization in network science focuses on understanding and optimizing the flow of information, influence, or behaviors within a network. It involves studying various models, techniques, and strategies to enhance the spread of information, identify influential nodes, improve network resilience, and optimize information routing.
Summary
7개의 Real-world dataset 에 IM technique 적용 전 / 후 모델 퍼포먼스를 비교하며 가설을 검증합니다. static / temporal network 각각의 데이터를 가지고 시간에 따라 변하는 network topology 를 추론한 후 도출된 new network topology 에 IM technique 을 적용합니다. 여기에서 핵심은 IM technique 과 link prediction technique 에서 각각 적용하는 관점간의 다양한 조합이라고 할 수 있습니다.
Insight
Static & Temporal IM , IM 의 고질적인 문제를 어떻게 풀어나갔으며 link prediction task 에 어떻게 접목했는지 라는 ‘왜’ 에 대해 의문을 가지고 논문을 읽으시면 많은 도움이 되실거라 생각합니다.
Building Your Own Schema.org
[https://medium.com/@Tonyseale/building-your-own-schema-org-7600a90e690a]
국내에서는 드물죠. knowledge graph engineer라는 직무로 근무하고 계시는 Tony Seale 님이 작성하신 글입니다. 근본적으로 돌아가서 저희는 지식그래프를 왜 사용할까요? 제가 생각하기엔 저희가 모르고 있는 지식간의 연관성을 유추하기 위해 즉, 잠재적인 의미 와 직관적인 의미를 도출하고 표현하기 위해서 사용한다고 생각합니다. 저는 지식그래프를 KGAT 논문을 읽으면서 처음 접하게 되었는데요. 여기에서도 Knowledge graph 의 semantic information 을 추가 적용한 모델이 추론 성능에서 좋은 결과를 보일것이다 라는 가설을 검증하기 위해 KGAT 를 제안합니다. 쉽게 말해보자면, ‘칼’이라는 대상을 두고 요리사 , 사무라이 , 세공사 각각을 다르게 해석하는바를 예시로 들 수 있겠습니다. 각각 대상들이 ‘칼’을 다른 관점으로 접근하기에 그 관점을 semantic 으로 간주하고 , 추천시스템 분야에 접목을 한거죠. 이야기가 잠시 빠졌습니다.
다시 돌아와서, 추천 시스템으로 지식그래프에 대해 처음알게되었던 그때와 지금 지식그래프를 바라보는 관점은 데이터 간의 지식을 발견해준다 라는 큰 맥락은 유사하나, 어디서부터 ? 어떻게 ? 라는 작은 맥락은 많이 다릅니다. 단편적으로 그냥 SPARQL 으로 ‘잘’ 질의하면 되지 라는 이전의 관점과 다르게 현재는 SPARQL 질의 전 어떻게 스키마를 정의할 것인가? 라는 관점이 추가되었습니다. 바로 ‘온톨로지’설계 관점이 들어간거죠.
본 게시물에서는 그 설계에 대한 관점을 짧은 글에 잘 작성했습니다. 온톨로지를 어떻게 설계할 것인가? 라는 의문이 있으셨던 분들 늘 인터넷상 어려운 자료들 때문에 고생많으셨을텐데요. 본 포스팅 그리고 작성한 medium 연관 포스팅들이 많은 도움이 될 거라 자신합니다. 굉장히 유용한 자료들이 많이 담겨있기에 강력하게 추천드리는 포스팅입니다.
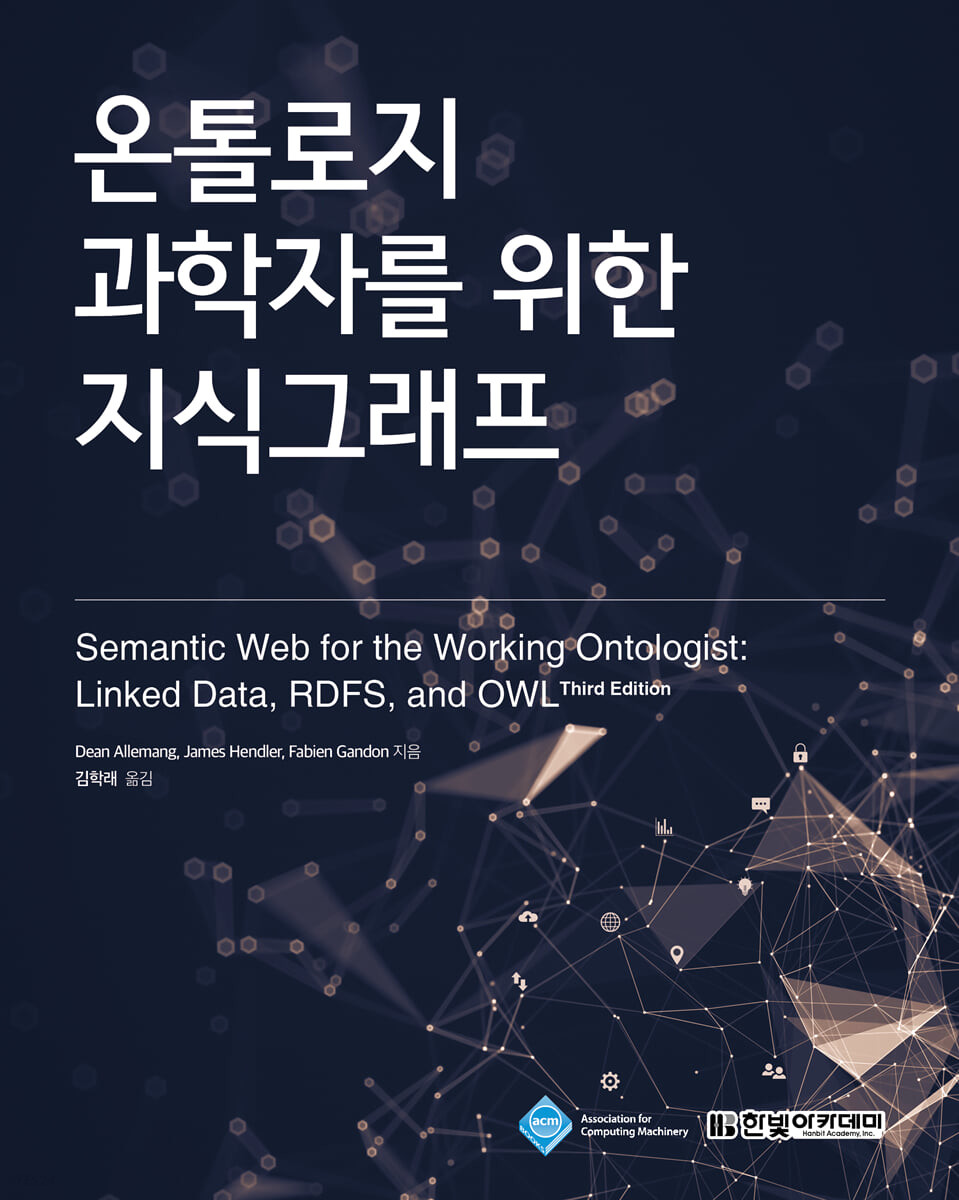
곁들어 보시면 좋을책입니다. 책 값이 비싸긴하지만, 안에 담겨있는 지식에 비하면 굉장히 저렴하다고 생각합니다. 지식그래프 & 온톨로지 해봤다! 하는 사람들과 대화하기위해 사전에 공부해야할 필독서라고 할 수 있습니다. 추천합니다.
[광고] GUG 첫 사이드 프로젝트가 완료되었습니다.
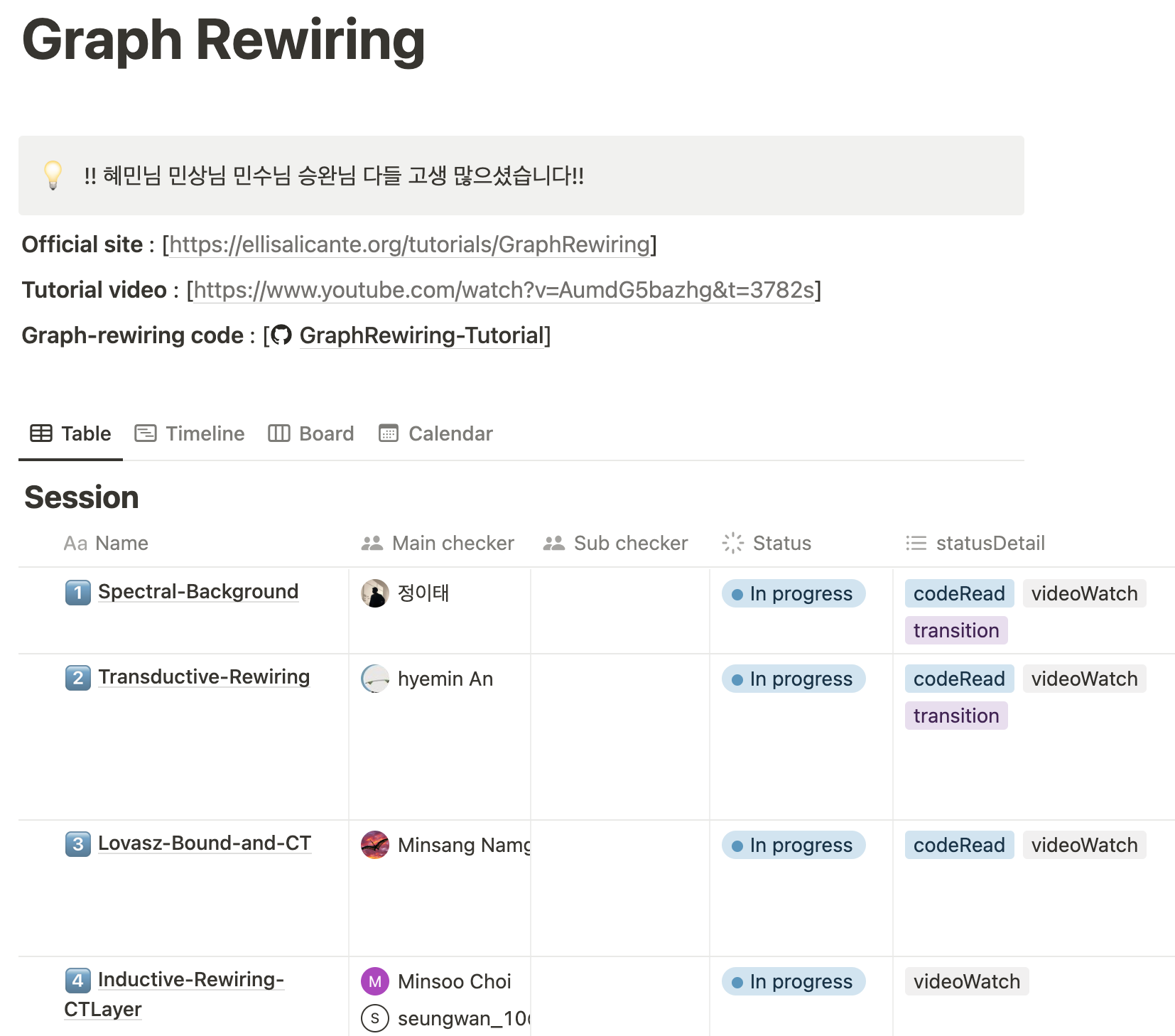
4월 30일경부터 7월 15일 근 2.5달간 매주 일요일마다 진행한 graph rewiring hands-on session 사이드 프로젝트가 마무리 되었습니다. 참석자 및 발표자 포함 총 10분가까이 참여해주셔서 graph rewiring 에 대한 지식을 공유 및 논의하는 자리로 끝을 잘 매듭지었다 라고 생각합니다. 토요일 주말 오전 150분 정도 진행된 세션간 참석자 및 발표자 모두 많은 배움이 있었을거라 믿어 의심치 않습니다.
이처럼, 그래프 관련 사이드 프로젝트를 기획하고 함께하고 싶으신 분들이 계시다면 GUG 채널을 활용해보시는건 어떠실까요? 자연어 , 비전 대비 상대적으로 마이너한 분야다보니 비슷한 관심사를 가진 분들을 뵙기엔 어렵습니다. 하지만, GUG 엔 다양한 분들이 많이 상주하고 계시기에 한층 프로젝트 진행하기에 수월하지 않을까 싶네요. 하단 디스코드 링크를 통해 접속할 수 있습니다.
** 오마카세 주인장이 매주 금요일 자체 그래프 해커톤을 기획하고 있으니, 관심있으신분들 참여 부탁드립니다 :)
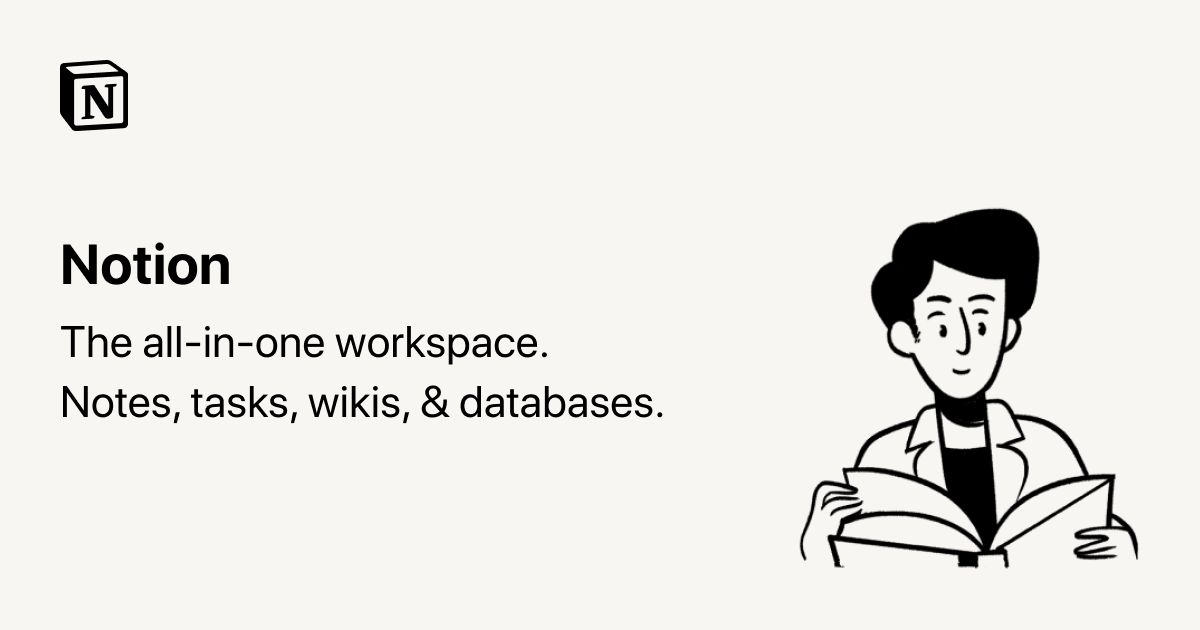